Research work in AI and mass spectrometry led by Arnaud Droit highlighted in «Nature Communications»
Arnaud Droit (Université Laval) and Frederic Precioso (Université Côte d’Azur) have designed an innovative method of artificial intelligence, referred to as cumulative learning, which makes it possible to learn a convolutional representation of data when the training set is small. The performance of the method, and its applicability to other fields led to a publication in the prestigious journal Nature Communications in November 2020.
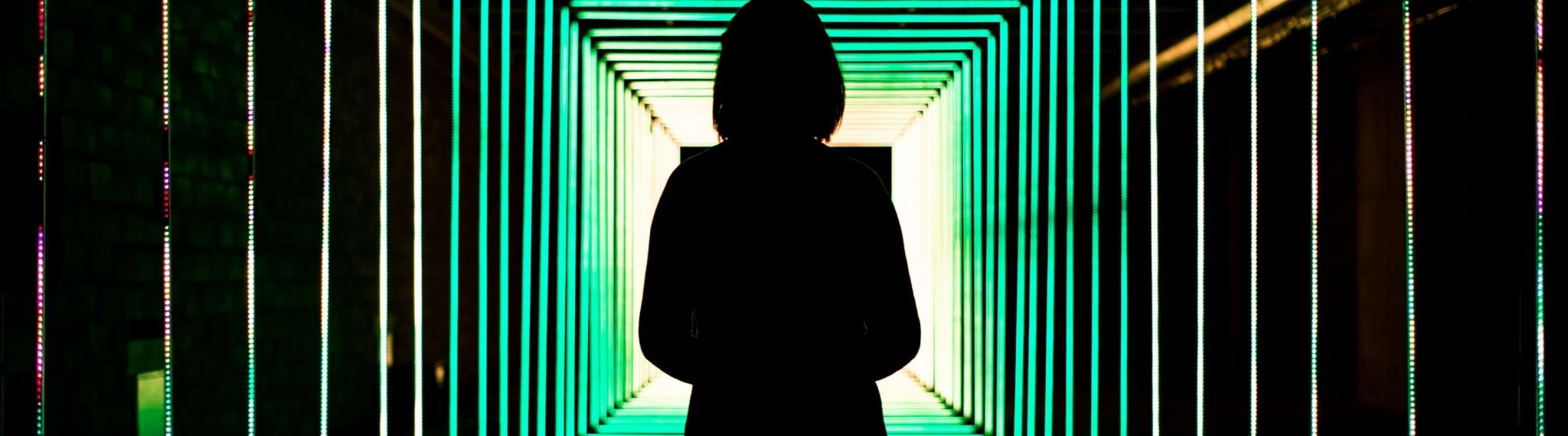
To evaluate their method and demonstrate its potential, they collaborated with a team from the PRISM laboratory at the University of Lille (U1192 INSERM) which is developing a mass spectrometry instrument for cancer detection. The difficulty of acquiring spectra does not allow the production of sufficient volumes of data to benefit from the advantages of deep learning.
Through cumulative learning, small numbers of spectra acquired for different types of cancer, on a variety of organs of various species, all contribute together to a deep learning representation that enables unparalleled results from the data available on the detection of targeted cancers.
The results of their research were published in the prestigious journal Nature Communications on November 5, 2020.
Frédéric Precioso is a university professor in the Laboratory of Computer Science, Signals and Systems of Sophia Antipolis (INRIA-CNRS-Université Côte d´Azur) and in the Maasai team (Inria). Arnaud Droit is an associate professor in the Department of Molecular Medicine in the Faculty of Medicine at Université Laval, and a researcher at the CHU de Québec-Université Laval Research Centre, where he heads the bioinformatics and proteomics platform. He is also a member of IID. This research work is the subject of the thesis of Khawla Seddiki, Ph.D. student in Prof. Arnaud Droit’s laboratory.
Read the article in Nature Communications
Read the press release from Université Côte-d’Azur regarding the publication

Abstract of the article
Rapid and accurate clinical diagnosis remains challenging. A component of diagnosis tool development is the design of effective classification models with Mass spectrometry (MS) data. Some Machine Learning approaches have been investigated but these models require time-consuming preprocessing steps to remove artifacts, making them unsuitable for rapid analysis. Convolutional Neural Networks (CNNs) have been found to perform well under such circumstances since they can learn representations from raw data. However, their effectiveness decreases when the number of available training samples is small, which is a common situation in medicine. In this work, we investigate transfer learning on 1D-CNNs, then we develop a cumulative learning method when transfer learning is not powerful enough. We propose to train the same model through several classification tasks over various small datasets to accumulate knowledge in the resulting representation. By using rat brain as the initial training dataset, a cumulative learning approach can have a classification accuracy exceeding 98% for 1D clinical MS-data. We show the use of cumulative learning using datasets generated in different biological contexts, on different organisms, and acquired by different instruments. Here we show a promising strategy for improving MS data classification accuracy when only small numbers of samples are available.
A privileged partnership between Université Côte d'Azur and Université Laval
This research work is the result of the privileged and dynamic partnership that has united Université Côte d’Azur and Université Laval since 2015 and which is based on a beneficial collaboration involving professors, researchers and students on both sides of the Atlantic.
Let’s keep in touch!
Would you like to be informed about IID news and activities? Subscribe now to our monthly newsletter.