Detection of Duplicates Among Non-structured Data From Different Data Sources
Présentation de David Beauchemin, étudiant à la maîtrise en informatique et membre du Groupe de recherche en apprentissage automatique de l’Université Laval (GRAAL), concernant la détection de doublons à l’intérieur de données non-structurées provenant de différentes sources.
Date
- 28 juillet 2020
Heure
15h00 à 16h00
Localisation
En téléprésence
Coûts
Gratuit
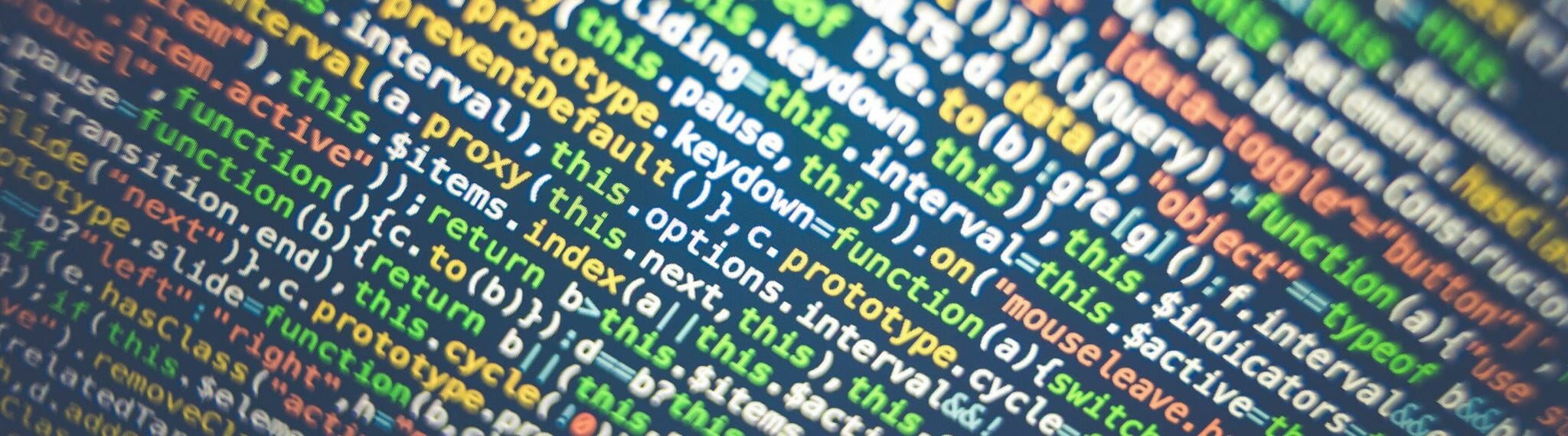
Résumé de la conférence (en anglais)
This work reports the exploration of two approaches to detecting duplicates between the companies descriptions in an internal database and those in an unstructured external source in commercial insurance. Since it is costly and tedious for an insurer to collect the information required to calculate an insurance premium, our motivation is to help them minimize the amount of resources necessary by extracting that information directly from external databases.
We first observed that the use of similarity algorithms allows us to detect most of the duplicates between databases using the name. Our experiments indicate that when the name is used as a source of comparison between the entities, a vast majority of these duplicates can be identified. Similar experiments, but using the address this time, allowed us to observe that it was also possible to identify duplicate companies by this feature, but to a lesser extent. Subsequently, we trained machine learning models to match duplicate companies using the name and the address at the same time. It is with these models that we observed the best results. In a final attempt to further improve our results, we used the N most likely entities to be a duplicate of a company, instead of only the first one, thus maximizing the recall to 91.07%.
Conférenciers : David Beauchemin, étudiant à la maîtrise en informatique, membre du Groupe de recherche en apprentissage automatique de l’Université Laval (GRAAL)
Restons en contact!
Vous souhaitez être informé des nouvelles et activités de l'IID? Abonnez-vous dès maintenant à notre infolettre mensuelle.