Predicting COVID-19 Intervention Outcome: A Québec Study
Présentation de l’étudiant au doctorat en physique, Daniel Gourdeau, portant sur l’utilisation de technologies liées à l’IA pour soutenir le personnel soignant dans la prise de décision concernant l’intubation des patients et patientes atteint.e.s de COVID-19.
Date
- 21 juillet 2020
Heure
15h00 à 16h00
Localisation
En téléprésence
Coûts
Gratuit
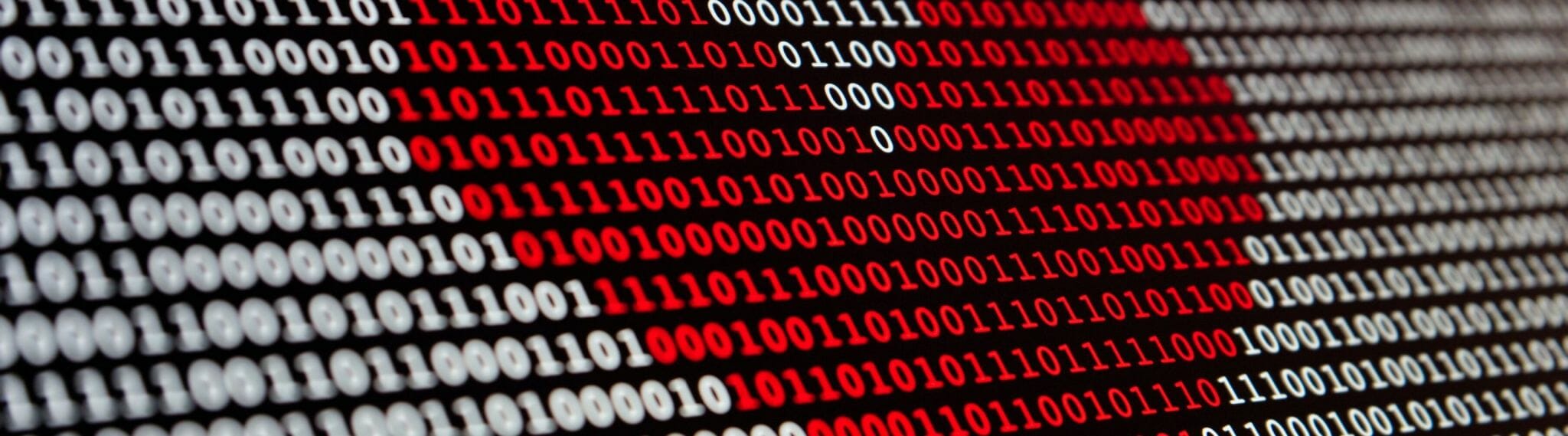
Résumé de la conférence (en anglais)
Severely infected COVID-19 patients that require admission to intensive care units (ICU) may need weeks of endotracheal intubation and mechanical ventilation to have a chance to recover. In a resource-constrained environment, these ventilation units have to be allocated to those patients with the highest chance of positive outcome.
Quebec Health Ministry triage guidelines have been issued for an extreme pandemic situation and laid down such decision rules for the allocation of mechanical ventilation. In the guidelines, the first level of triage prioritize care to patients with less than a 80% expected mortality rate, 50% at the second level of triage,and 30% at the last level. However there is no accepted test to date that can determine the outcome (i.e. discharge or mortality) for ventilation in COVID-19.
In order to help with these decisions, we propose to provide accurate, quantitative predictive information on outcome from the analysis of bedside chest X-ray exams (CXR), which have been recommended for prognosis evaluation in COVID-19. We first tuned a DenseNet121 pre-trained on ImageNet on CheXpert, a large dataset of CXR with various clinical presentations (223 414 CXR, 14 radiological signs), then used it as a feature extractor to predict the outcome of ventilation on ICU patients. A dataset of 53 intubated COVID-19 patients was collected from two Quebec hospitals. 34 of these patients were used in the training set and results are reported using leave-patient-out cross-validation. The remaining 19 patients will constitute the testing set once model development is complete. The features extracted by the DenseNet121 can be used to predict mortality with a 0.77 AUC, comparable to the 0.85 AUC obtained using age and sex, which are strong predictors of mortality. Combining these two types of features leads to an even stronger classifier with a 0.90 AUC.
We are currently collecting more data and incorporating more clinical variables such as vitals signs and risk factors to improve our models.
Conférencier : Daniel Gourdeau, étudiant au doctorat en physique, Université Laval
Restons en contact!
Vous souhaitez être informé des nouvelles et activités de l'IID? Abonnez-vous dès maintenant à notre infolettre mensuelle.