Journée sur l’équité et la discrimination en assurance 2024
16 mai 2024, 8h30 à 17h | En mode hybride
En direct de l’Université Laval, pavillon Alexandre-Vachon, local 2880 + Zoom
Workshop on Fairness and Discrimination in Insurance 2024
May 16th 2024, 8:30AM – 5PM | Hybrid Event
Live from Université Laval, pavillon Alexandre-Vachon, room 2880 + Zoom
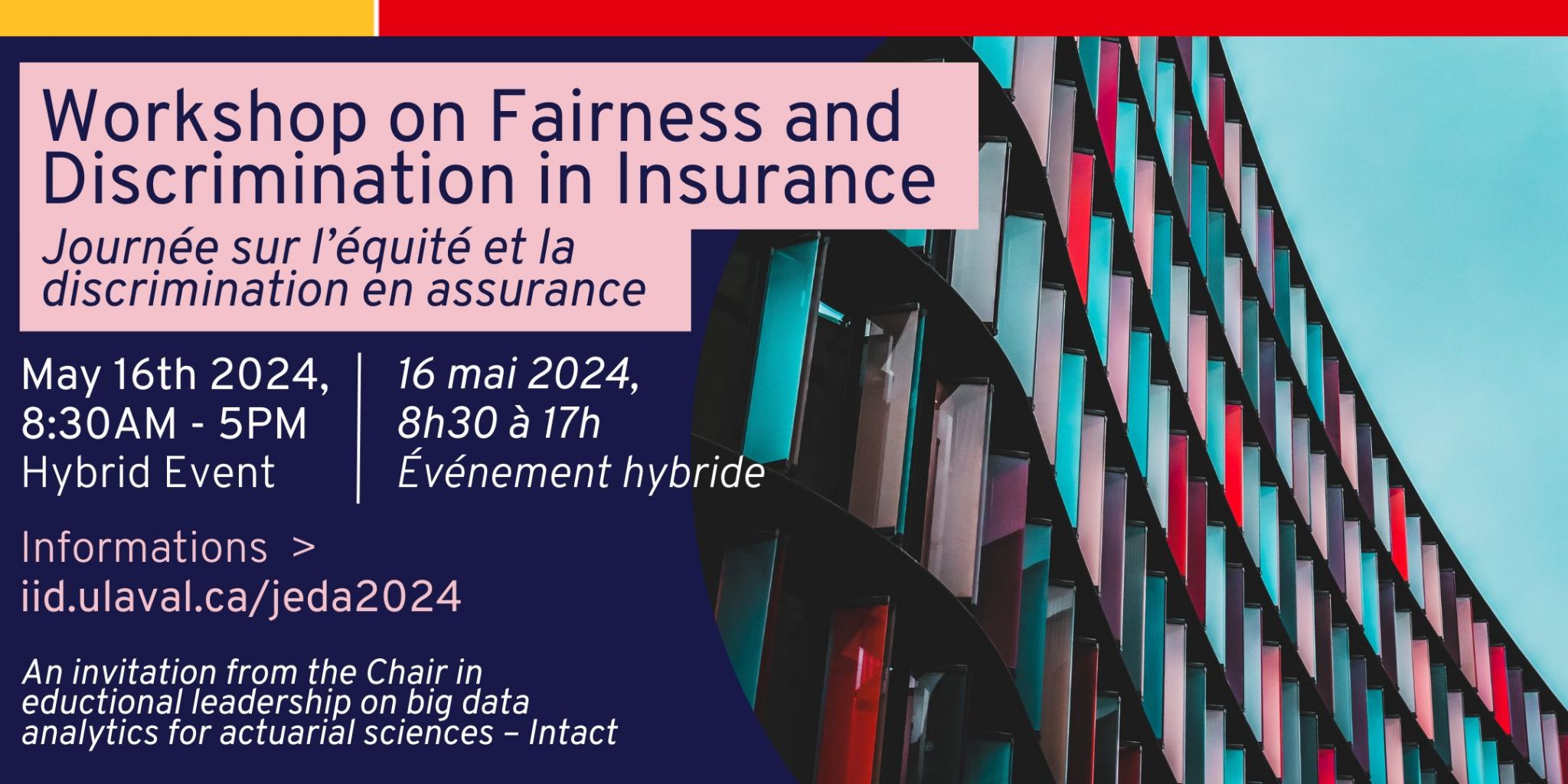
Cette journée sur l’équité et la discrimination en assurance aura lieu simultanément en ligne et à l’Université Laval le 16 mai 2023. Les présentations seront en anglais.
Avec un heureux mélange de présentateurs de l’industrie, du milieu académique et d’associations professionnelles, de multiples présentation d’experts autour de cette thématique d’importance vous seront proposées. Que vous soyez un praticien dans l’industrie de l’assurance, un étudiant ou un chercheur en actuariat, ce workshop est pour vous!
Une invitation de la Chaire de Leadership en enseignement en analyse de données massives pour l’actuariat – Intact de l’Université Laval. L’événement est présenté avec l’appui du Laboratoire de mathématique actuarielle et financière Quantact du Centre de recherche en mathématiques.
This day on fairness and discrimination in insurance will take place simultaneously online and at Université Laval on May 16, 2023.
With a happy mix of presenters from industry, academia and professional associations, you’ll be able to enjoy a wide range of expert presentations on this important topic. Whether you’re a practitioner in the insurance industry, a student or a researcher in actuarial science, this workshop is for you!
An invitation from Université Laval’s Chair in eductional leadership on big data analytics for actuarial sciences – Intact. The event is presented with the support of the Quantact Actuarial and Financial Mathematics Laboratory of the Centre de recherche en mathématiques.
Programme de l'événement | Event Schedule
Morning
9:00
Introductory Remarks
Marie-Pier Côté, Université Laval
9:15
Fairness-Aware Insurance Pricing: Principles, Fairness Criteria and Welfare Implications
Fei Huang, UNSW Sidney
10:20
Coffee Break
10:35
Practitioner View of US Regulation on Fairness
David Schraub, Chicago Actuarial Association
11:20
Issues and Discussion: Best Practices for the Responsible Use of AI in the Financial Sector
Emmanuel Hamel, Autorité des marchés financiers
12:00
Lunch (included)
Afternoon
13:15
Rethinking Insurance Fairness in the Wake of Climate Change
Laurence Barry, Chaire PARI
14:15
EquiPy: A Python Package for Sequential Fairness using Optimal Transport with Applications in Insurance
Agathe Fernandes Machado, UQÀM
14:40
Coffee Break
15:00
Practical Applications of Bias Measurement and Mitigation Techniques in Insurance Rating: A Preview of CAS Research
Mallika Bender, Casualty Actuarial Society
15:40
Exploring the Intersection Between Actuarial and Social Fairness
Christopher Cooney, TD Insurance
16:20
A Fair Price to Pay: Exploiting Causal Graphs for Fairness in Insurance
Olivier Côté, Université Laval
16:45
Closing Remarks
Marie-Pier Côté, Université Laval
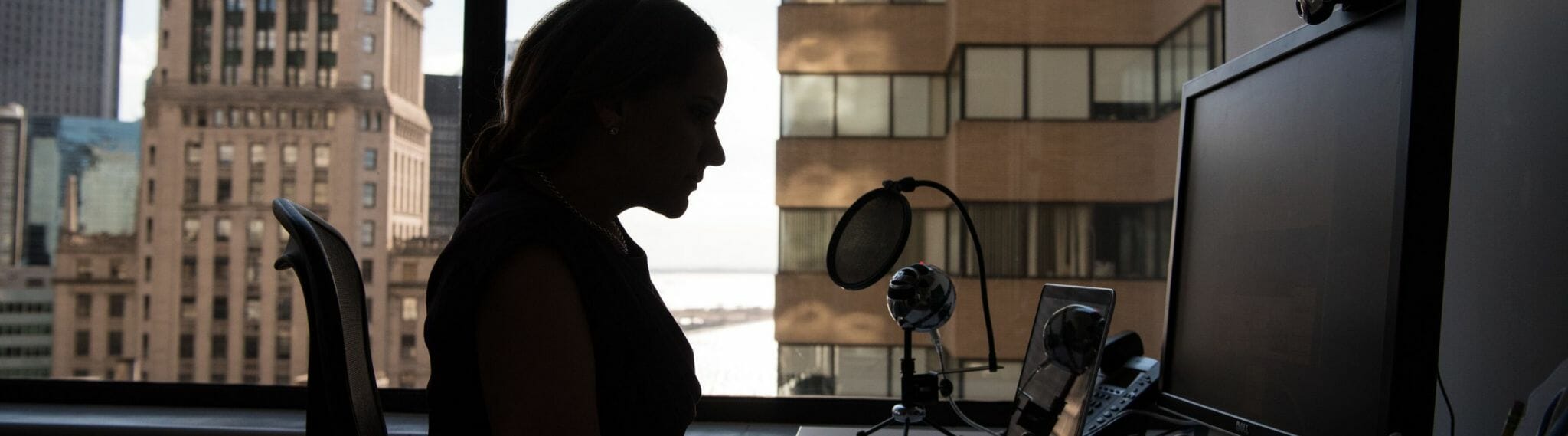
Conférencières principales | Keynote Speakers
Laurence Barry (Chaire PARI)
Laurence Barry is a graduate of the Ecole Polytechnique, a statistician and economist from ENSAE and a qualified actuary. She also holds a PhD in political science. She is currently co-holder of the PARI Chair (Programme de recherche sur l’Appréhension des Risques et des Incertitudes), and is involved in both academic and actuarial consulting projects. Her research focuses on the transformations of modern rationality and, more recently, its algorithmic mutation.
Ancienne élève de l’Ecole polytechnique, statisticienne économiste de l’ENSAE et actuaire qualifiée, Laurence Barry est également titulaire d’un doctorat de sciences politiques. Elle est aujourd’hui co-titulaire de la chaire PARI (Programme de recherche sur l’Appréhension des Risques et des Incertitudes) et mène de front des projets académiques et de conseil en actuariat. Ses recherches portent sur les transformations de la rationalité moderne et plus récemment sa mutation algorithmique.
Fei Huang (UNSW Sydney)
Fei Huang is a Senior Lecturer in Risk and Actuaries Studies and Lead – Data and AI Tech at UNSW Business AI Lab. She received her BSc. in Mathematics from Xiamen University, MPhil in Actuarial Science from the University of Hong Kong, and PhD in Actuarial Studies from the Australian National University. Her research focuses on Ethical AI and Data Science for Insurance. In particular, Fei Huang studies insurance discrimination and pricing fairness and develops statistical learning methods for various actuarial applications. She has published in top-tier actuarial journals and received the Carol Dolan Actuaries Summit Prize and ASTIN Colloquium Best Paper Award for her research on anti-discrimination insurance pricing.
Fei Huang est Professeure assistante en risque et études actuarielles et Cheffe – données et technologie IA à UNSW Business AI Lab. Elle détient un baccalauréat en mathématiques de Xiamen University, une maitrise en sciences actuarielles de l’University of Hong Kong et un doctorat en études actuarielles de l’Australian National University. Ses recherches portent sur l’intelligence artificielle éthique et la science des données en assurance. En particulier, Fei Huang étudie la discrimination en assurance et la tarification équitable et développe des méthodes d’apprentissage statistique pour des fins actuarielles. Elle a publié dans des revues actuarielles de haut niveau et sa recherche sur la tarification sans discrimination en assurance a été reconnue par le Carol Dolan Actuaries Summit Prize et le ASTIN Colloquium Best Paper Award.
Conférenciers et conférencières | Speakers
Mallika Bender (Casualty Actuarial Society)
Mallika Bender is a Fellow of the Casualty Actuarial Society (CAS) and the Diversity, Equity & Inclusion (DE&I) Staff Actuary for the CAS, where she leads initiatives to enhance diversity within the profession and manages the CAS Approach to Race and Insurance Pricing. Prior to joining the CAS, she spent thirteen years in traditional Property & Casualty actuarial roles performing a range of functions, including pricing, reserving and predictive modeling, in support of Personal, Commercial and Specialty insurance lines in the United States and Australia. Mallika holds a Bachelor of Science in Applied Statistics from American University and lives in Philadelphia, PA.
Mallika Bender est Fellow de la Casualty Actuarial Society (CAS). Elle est l’actuaire en charge de l’équité, la diversité et l’inclusion pour la CAS, où elle mène des initiatives pour renforcer la diversité dans la profession et gère la CAS Approach to Race and Insurance Pricing. Avant de rejoindre la CAS, elle a travaillé pendant treize ans en assurance IARD sur des tâches actuarielles variées incluant la tarification, les réserves, l’analyse prédictive, pour des branches d’activités personnelles, commerciales ou de spécialité, aux États-Unis et en Australie. Mallika Bender détient un baccalauréat en statistique appliquée de l’American University et habite à Philadelphie.
Christopher Cooney (TD Insurance)
Christopher Cooney joined TD Insurance in March 2016 as the Vice President, Pricing, for TD Insurance’s General Insurance (i.e., Home and Auto) Products, and presently supports the insurance business as the VP, Analytics and Modelling. Formerly the Vice President, Pricing / Corporate Underwriting / Insurance Information Services at RBC Insurance where he worked for 18 years, Chris has been responsible for the pricing of direct-to-consumer home and auto insurance products for over 25 years. He is a Fellow of the Canadian Institute of Actuaries (CIA) and of the Casualty Actuarial Society. In the fairness realm, Chris supported the development of the CIA’s « Big Data and Risk Classification » public statement released in July 2022. This document articulated many of the social and economic implications associated with sensitive pricing and underwriting data elements. He also serves as Past-Chair of the Facility Association Board of Directors, and as the Chair of the Industry Advisory Committee at GISA.
Christopher Cooney a rejoint TD Insurance en mars 2016 comme Vice-président, tarification pour les produits d’assurance générale de TD Insurance, et offre présentement son soutien aux activités d’assurance comme VP, analytique et modélisation. Précédemment le Vice President, Pricing / Corporate Underwriting / Insurance Information Services à RBC Insurance où il a travaillé pendant 18 ans, Chris a été responsable de la tarification pour les produits directs d’assurance auto et habitation pour plus de 25 ans. Il est Fellow de l’Institut canadien des actuaires (ICA) et de la Casualty Actuarial Society. Dans le monde de l’équité, Chris a participé au développement de l’énoncé public « Big Data and Risk Classification » de l’ICA publié en juillet 2022. Ce document a formulé plusieurs des implications sociales et économiques associées avec des données sensibles pour la tarification et la souscription. Il a aussi servi comme président sortant de la Facility Association Board of Directors et comme président de l’Industry Advisory Committee de GISA.
Marie-Pier Côté (Université Laval)
Marie-Pier Côté is an associate professor at the School of Actuarial Science at Université Laval. She holds the Chair in educational leadership on big data analytics for actuarial sciences – Intact. Prior to joining Laval, she completed a Master’s and a PhD in statistics at McGill University under the supervision of Christian Genest. She is a fellow of the Society of Actuaries and an associate of the Canadian Institute of Actuaries. Her research interests lie in statistical learning, fairness and copula models for general insurance applications.
Marie-Pier Côté est professeure agrégée à l’École d’actuariat de l’Université Laval. Elle est titulaire de la Chaire de leadership en enseignement en analyse de données massives pour l’actuariat – Intact et co-organise cet évènement avec Arthur Charpentier (UQAM). Avant de se joindre à l’Université Laval, Marie-Pier Côté a complété ses études de maitrise et de doctorat en statistique à l’Université McGill, sous la supervision de Christian Genest. Elle est membre fellow de la Society of Actuaries et associée de l’Institut canadien des actuaires. Ses intérêts de recherche sont l’apprentissage statistique, l’équité et les modèles de copules pour l’assurance générale.
Olivier Côté (Université Laval)
Olivier Côté is a Ph.D. student in Actuarial Science at Université Laval. His thesis focuses on fairness in insurance, conducted in collaboration with one of Canada’s largest property and casualty insurers and supervised by professors Marie-Pier Côté (Université Laval) and Arthur Charpentier (Université du Québec à Montréal). Olivier has received several scholarships highlighting his teaching excellence, academic excellence, and leadership both in academia and sports.
Olivier Côté est doctorant en actuariat à l’Université Laval. Sa thèse sur l’équité dans l’assurance est réalisée en collaboration avec l’un des plus grands assureurs de dommages au Canada. Il est supervisé par les professeurs Marie-Pier Côté (Université Laval) et Arthur Charpentier (Université du Québec à Montréal). Olivier a reçu plusieurs reconnaissances soulignant son excellence dans l’enseignement, son excellence académique, et son leadership tant dans le domaine universitaire que dans le sport.
Agathe Fernandes Machado (UQÀM)
Agathe Fernandes Machado is a PhD student in mathematics at the Université du Québec à Montréal (UQAM) whose research focuses on the fairness of Machine Learning algorithms and the analysis of the uncertainty of these models through the concept of calibration, with an emphasis on their application in the field of insurance. Before joining UQAM, she pursued an academic path in France, where she obtained a master’s degree from IMT Atlantique, specializing in mathematical and computational engineering methods, particularly in the field of Machine Learning, as well as a master’s degree in actuarial science, with a particular interest in modeling risks associated with natural disasters.
Agathe Fernandes Machado est étudiante au doctorat en mathématiques à l’Université du Québec à Montréal (UQAM). Sa recherche se focalise sur l’équité des algorithmes d’apprentissage automatique et l’analyse de l’incertitude de ces modèles à travers le concept de calibration, avec un accent sur leur application dans le domaine de l’assurance. Avant de rejoindre l’UQAM, elle a suivi un parcours académique en France, où elle a obtenu une maîtrise à l’IMT Atlantique, se spécialisant dans les méthodes d’ingénierie mathématique et informatique, notamment dans le domaine de l’apprentissage automatique. Elle est aussi titulaire d’un master en actuariat, lors duquel elle a étudié la modélisation des risques associés aux catastrophes naturelles.
David Schraub (Chicago Actuarial Association)
David Schraub is a consultant with 25 years of actuarial experience, bringing the actuarial lens to innovation and technology for the insurance industry; he advises clients on how to implement artificial intelligence use cases. With a solid technical education from the prestigious Ecole Nationale de la Statistique et de l’Administration Economique master’s degree in actuarial science, David Schraub continues to lead and support the industry by chairing the Artificial Intelligence subcommittee of the American Academy of Actuaries, and being the president of the Chicago Actuarial Association. He also volunteers on Behavioral Economics and Data Science and Analytics committees, as well as on the project oversight group of the Society of Actuaries Quantitative Fairness Metrics project. These activities keep him on the leading edge of how the family of Predictive Modeling techniques impacts the industry, from the old GLM-GAM to AI, ML and newer GenAI, LLM techniques.
David Schraub est un consultant avec 25 ans d’expérience en actuariat. Il amène un point de vue actuariel sur l’innovation et la technologie dans l’industrie de l’assurance ; il conseille ses clients sur la façon d’implémenter l’intelligence artificielle dans leurs processus. Avec une formation solide de maitrise en actuariat de la prestigieuse Ecole Nationale de la Statistique et de l’Administration Economique, David Schraub continue de guider et aider l’industrie en présidant le sous-comité sur l’intelligence artificielle de l’American Academy of Actuaries et la Chicago Actuarial Association. Il est bénévole sur les comités Behavioral Economics et Data Science and Analytics, et sur le groupe de supervision de projets du Quantitative Fairness Metrics project de la Society of Actuaries. Ces activités le gardent à la fine pointe sur la façon dont les techniques de modélisation prédictive influencent l’industrie, lors du passage des GLM-GAM traditionnels vers l’IA, l’apprentissage automatique, et vers les récentes techniques d’intelligence artificielle générative et des grands modèles de langage.
Emmanuel Hamel (Autorité des marchés financiers)
Emmanuel Hamel is a data scientist in the research lab of the Autorité des marchés financiers (AMF), the regulator of the financial market in the province of Québec. As a data scientist, Emmanuel works on projects to increase the efficiency of the AMF (e.g., calibrate mathematical models, create artificial intelligence models, visualize data, combine datasets). Emmanuel is also a leader of the R, Python and PowerBI communities in the AMF. He is also responsible to set up research projects with universities that are useful for the AMF and the financial industry. Emmanuel has been working at the AMF for 13 years, first as a quantitative modeler. Also, Emmanuel is a lecturer at the Université Laval in Québec. Emmanuel graduated from the Université Laval and holds a PhD in actuarial science. His main research interests are financial guarantees in actuarial science and stochastic processes. Emmanuel is involved in different research teams and projects on his personal time.
Emmanuel Hamel est un scientifique de données au sein du laboratoire de recherche de l’Autorité des marchés financiers (AMF), l’organisme de réglementation du marché financier au Québec. En tant que scientifique de données, Emmanuel travaille sur des projets visant à augmenter l’efficacité de l’AMF (par exemple, calibrer des modèles mathématiques, créer des modèles d’intelligence artificielle, visualiser des données, combiner des ensembles de données). Emmanuel est également un leader des communautés R, Python et PowerBI au sein de l’AMF. Il est également chargé de mettre en place des projets de recherche, avec les universités, qui sont utiles à l’AMF et à l’industrie financière. Emmanuel travaille à l’AMF depuis 13 ans, il occupait au départ un poste de modélisateur quantitatif. Il est également chargé de cours à l’Université Laval à Québec. Emmanuel est diplômé de l’Université Laval et titulaire d’un doctorat en actuariat. Ses principaux intérêts de recherche sont les garanties financières en actuariat et les processus stochastiques. Emmanuel fait partie de différents projets et équipes de recherche pendant ses temps libres.
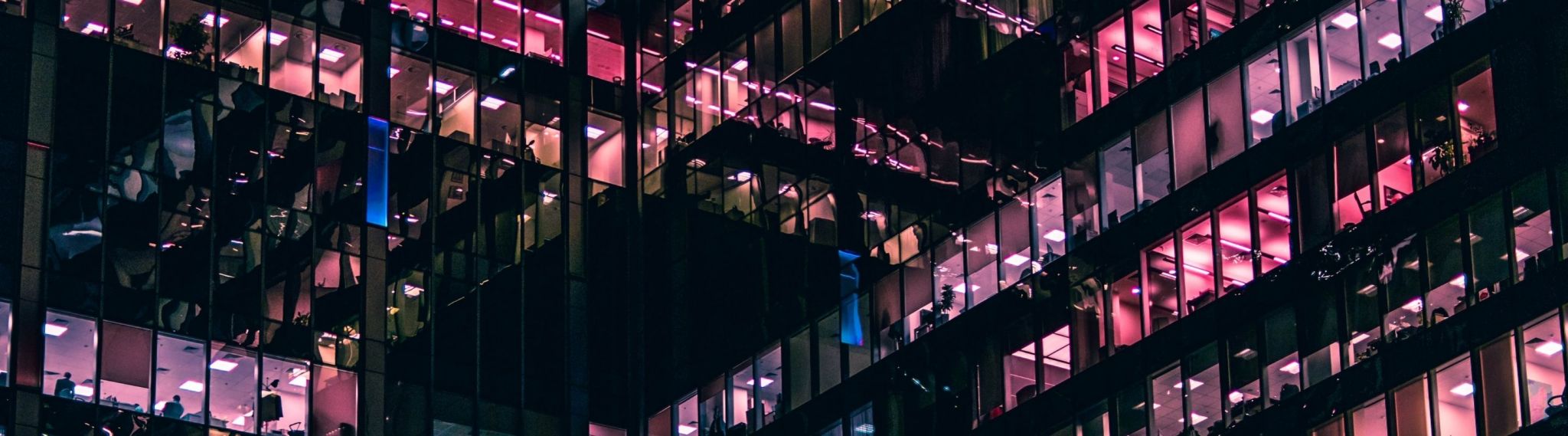
Résumé des conférences | Abstracts
Fairness-aware Insurance Pricing: Principles, Fairness Criteria, and Welfare Implications
Tarification consciente de l’équité en assurance : principes, critères d’équité et conséquences sur le bien-être
Fei Huang
In this presentation, I will focus on answering three research questions related to fairness-aware insurance pricing: (1) What are the social and economic principles that can be used to assess the appropriateness of insurance discrimination? (2) How can we match the existing and potential anti-discrimination insurance regulations/policies with fairness criteria (mostly developed in the machine learning community) and develop anti-discrimination insurance pricing models? (3) What are the welfare implications of existing and potential fair insurance pricing regulations/policies on both consumers and firms? A list of open questions to be answered and related interpretation/transparency challenges will be discussed at the end of this presentation.
Dans cette présentation, je vais répondre à trois questions sur la tarification d’assurance consciente de l’équité : (1) Quels sont les principes sociaux et économiques pouvant être utilisés pour évaluer si la discrimination en assurance est appropriée ? (2) Comment pouvons-nous apparier les lois anti-discrimination existantes et possibles avec les critères d’équité (développés par les chercheurs en apprentissage automatique) et construire des modèles de tarification équitable en assurance? (3) Quels sont les effets des réglementations de tarification anti-discrimination sur le bien-être des consommateurs et des compagnies? Une liste de questions ouvertes auxquelles il faut répondre et les problèmes d’interprétation et de transparence qui en découlent seront abordés à la fin de la présentation.
Rethinking Insurance Fairness in the Wake of Climate Change
Repenser l’équité en assurance dans le contexte des changements climatiques
Laurence Barry
The recent withdrawal of insurers from certain regions and risks in the United States reopens the question of “the what and how” of insurance fairness: is it inevitable that the increasing frequency of natural disasters is accompanied by a decrease in insurance coverage, leaving more and more vulnerable people exposed to such damage? In this presentation, I argue that this fatalistic perception depends on the adoption of a specific economic vision of what insurance is, and that climate change may prompt us to reconsider this vision. In the first part, I will present a Foucauldian approach to insurance mechanisms, highlighting the tension between an individualistic and a solidary conception of insurance fairness, in relation to different types of governmentalities, and concluding by proposing an alternative approach: could natural disaster insurance be seen as an Ostromian common? The second part of the presentation will explore these questions through examples of protection schemes adopted in different countries.
Le retrait récent des assureurs de certaines régions ou risques aux États-Unis relance la question sur les objectifs et les méthodes d’équité en assurance : est-il inévitable que la fréquence croissante des catastrophes naturelles s’accompagne d’une diminution de la couverture d’assurance, laissant de plus en plus de personnes vulnérables exposées à de tels dommages ? Dans cette présentation, je soutiens que cette perception fataliste dépend de l’adoption d’une vision économique spécifique de ce qu’est l’assurance, et que les changements climatiques peut nous inciter à reconsidérer cette vision. Dans la première partie, je présenterai une approche foucaldienne des mécanismes d’assurance, en soulignant la tension entre les conceptions individualiste et solidaire de l’équité de l’assurance, en relation avec différents types de gouvernementalité, et je conclurai en proposant une approche alternative : l’assurance contre les catastrophes naturelles pourrait-elle être considérée comme un commun ostromien ? La deuxième partie de la présentation explorera ces questions à travers des exemples de régimes de protection adoptés dans différents pays.
A Fair price to pay: exploiting causal graphs for fairness in insurance
Le juste prix: une perspective causale sur l’équité dans l’assurance
Olivier Côté
In many jurisdictions, insurance companies must not discriminate on some given policyholder characteristics. Omission of prohibited variables from models prevents direct discrimination, but fails to address proxy discrimination, a phenomenon especially prevalent when powerful predictive algorithms are fed with an abundance of acceptable covariates. Given the insurance industry’s reliance on personal data and its major role in society, insurance companies ought to be wary about unfairness towards their insureds. Exploiting causal graphs, we discuss potential sources of bias, formally define direct and indirect discrimination, and study the properties of fairness methodologies. A novel categorization of fair methodologies into five families (best-estimate, unaware, aware, hyperaware, and corrective) is constructed based on their expected fairness properties.
Sur de nombreux territoires, les compagnies d’assurance ne peuvent pas discriminer sur la base de certaines caractéristiques des titulaires de polices. L’omission des variables interdites des modèles prévient la discrimination directe, mais ne parvient pas à éradiquer la discrimination par proxy, un phénomène particulièrement répandu lorsque des algorithmes prédictifs puissants sont alimentés avec une abondance de variables acceptables. Les assureurs, s’appuyant fortement des données personnelles, doivent particulièrement veiller à l’équité entre les assurés. En exploitant les graphes causaux, nous discutons des sources potentielles de biais, définissons formellement la discrimination directe et indirecte, et étudions les propriétés des méthodologies d’équité. Une nouvelle catégorisation des méthodologies équitables en cinq familles (prime précise, inconsciente, consciente, hyperconsciente et réparatrice) est construite sur la base de leurs propriétés d’équité attendues.
Practitioner view of US regulations on fairness
Les réglementations américaines sur l’équité : perspective d’un praticien
David Schraub
Are you prepared for the new regulations that are going to affect how you market, price and underwrite with predictive analytics? It will also affect how you detect fraud and perform other internal controls. On one end you can gain or lose competitive advantage, on the other end you can run into major liability with lawmakers, regulators and with the court system. Let’s see how to implement these new tools the right way to detect fraud, identify target market, and anticipate policyholder behavior. David Schraub will share key insights on the upcoming US regulations, on how companies are preparing for these requirements, and make a compare and contrast with regulations in other jurisdictions as appropriate.
Êtes-vous prêt pour la nouvelle réglementation qui affectera comment vous vendez, tarifez et souscrivez les risques avec l’analyse prédictive? La façon dont vous détectez les frauds, et autres modèles internes, seront aussi influencés par ces règles. D’un côté, vous pourriez gagner ou perdre votre avantage compétitif, de l’autre, vous pourriez vous créer de gros problèmes avec les législateurs, les organismes de réglementation et le système judiciaire. Penchons-nous sur la bonne façon d’implémenter ces nouveaux outils pour détecter les fraudes, identifier le marché cible, et anticiper le comportement des titulaires de police. David Schraub partagera ses constats cruciaux sur les futures réglementations américaines, sur la façon dont les compagnies se préparent pour ces exigences, et fera une comparaison avec les réglementations en vigueur dans d’autres juridictions.
Exploring the intersection between Actuarial and Social Fairness
Exploration de l’intersection entre l’équité actuarielle et sociale
Christopher Cooney
Insurance underwriting and ratemaking are critical insurance functions with a dependency upon collection and analysis of wide sets of data. Within these datasets, however, pricing actuaries often find themselves at the intersection of complex issues that require a balance between actuarial fairness, and social equity. In July 2022, the Canadian Institute of Actuaries published its policy statement « Big Data and Risk Classification » document which discusses these complexities and offers an opinion regarding the need to approach data usage restrictions with caution. As a member of the working group developing the statement, the presenter will also share experiences and perspectives from over 25 years working with regulators and policy makers from within the Canadian personal lines insurance industry.
La souscription des risques et la tarification sont des tâches critiques en assurance qui dépendent de la collection et l’analyse de grands jeux de données. Dans ces jeux de données, toutefois, les actuaires en tarification se trouvent à l’intersection de questions complexes qui demandent de trouver l’équilibre entre l’équité actuarielle et sociale. En juillet 2022, l’Institut canadien des actuaires a publié son document intitulé « Big Data and Risk Classification », qui traite de ces problèmes complexes et offre une opinion sur le besoin d’approcher l’utilisation des données avec précautions. Comme membre du groupe de travail qui a rédigé cet énoncé, le conférencier partagera ses perspectives tirées de ses 25 ans d’expérience de travail avec les organismes de réglementation et les décideurs au Canada pour les branches d’activités personnelles en assurance.
Practical Applications of Bias Measurement and Mitigation Techniques in Insurance Rating: A Preview of CAS Research
Applications pratiques des métriques et des techniques d’atténuation des biais dans la tarification en assurance: un aperçu de la recherche de la CAS
Mallika Bender
This session will preview one of the five new research projects currently in progress as part of Phase 2 of the CAS Approach to Race and Insurance Pricing. Practical Approaches to Bias Measurement and Mitigation in Insurance Rating is designed as a practitioner’s guide that may support actuaries as they initiate fairness analyses in their work. We will begin by discussing the application of variations on the BIFSG method for imputing protected class information when actual data is not readily available. Then we will review several potential fairness metrics and bias detection analyses and mitigation techniques to insurance rating, along with the advantages and limitations of each approach. This session will also provide a sneak peek of other Race and Insurance Pricing research projects underway.
Cette session présentera un aperçu de l’un des cinq nouveaux projets de recherche en cours dans le cadre de la deuxième phase de la CAS Approach to Race and Insurance Pricing. L’article présenté, sur les approches pratiques de la mesure et de l’atténuation des biais dans la tarification en assurance, est conçu comme un guide du praticien qui peut aider les actuaires à entreprendre des analyses d’équité dans le cadre de leur travail. Nous commencerons par discuter de l’utilisation de variantes de la méthode BIFSG pour imputer des informations sur les classes protégées lorsque les données réelles ne sont pas facilement disponibles. Ensuite, nous passerons en revue plusieurs métriques potentielles d’équité, des analyses de détection des biais et des techniques d’atténuation pour la tarification d’assurance, ainsi que les avantages et les limites de chaque approche. Cette session donnera également un aperçu des autres projets de recherche en cours sur la race et la tarification de l’assurance.
EquiPy: A Python Package for Sequential Fairness using Optimal Transport with Applications in Insurance
EquiPy: Un package Python pour l’équité séquentielle basé sur le transport optimal et applications en assurance
Agathe Fernandes Machado
In certain regions of Canada and the United States, discrimination based on multiple sensitive attributes, such as race and gender, is prohibited for insurance pricing. While approaches have been proposed to address these biases in predictive systems, they often struggle to offer a clear and accurate pathway to fairness, especially when multiple sensitive variables are involved. In this context, I will introduce EquiPy, a new open-source Python package implementing sequential fairness across multiple sensitive attributes via Optimal Transport. EquiPy will be demonstrated through an illustrative example using insurance data.
Dans certaines régions du Canada et des États-Unis, la discrimination basée sur plusieurs attributs sensibles, tels que la race et le genre, est interdite pour la tarification en assurance. Bien que des approches aient été proposées pour traiter ces biais dans les systèmes prédictifs, elles ont souvent du mal à offrir un chemin clair et précis vers l’équité, surtout lorsque plusieurs variables sensibles sont impliquées. Dans ce contexte, j’introduirai EquiPy, un nouveau package Python open-source, mettant en œuvre l’équité séquentielle au regard de plusieurs attributs sensibles via le Transport Optimal. EquiPy sera présenté à travers un exemple illustratif utilisant des données d’assurance.
Issues and Discussion: Best Practices for the Responsible Use of AI in the Financial Sector
Réflexion et discussion : meilleures pratiques pour l’utilisation responsable de l’IA dans le secteur financier
Emmanuel Hamel
The Autorité des marchés financiers (AMF) is a key player within Québec’s financial ecosystem. In its role as regulator, the AMF acts to maintain a financial sector that is dynamic, operates with integrity and warrants public confidence. The AMF sets the rules and standards governing market activities and supports the adoption of best practices. The AMF has undertaken various mission-aligned initiatives relating to the digital transformation of the financial sector, in particular, to anticipate regulatory and consumer protection issues. The AMF has a strong interest in the responsible use of artificial intelligence (AI) and is actively reflecting about this subject. The AMF recently published a paper which outlines best practices for the responsible use of AI in the financial sector. An overview of the best practices will be presented.
L’Autorité des marchés financiers (AMF) est un acteur clé de l’écosystème financier québécois. Dans son rôle de régulateur, l’AMF agit afin de maintenir un secteur financier dynamique, intègre et digne de la confiance du public. L’AMF établit les règles et les normes qui régissent les activités du marché et soutient l’adoption de bonnes pratiques. L’AMF a entrepris diverses initiatives, conformes à sa mission, concernant la transformation numérique du secteur financier afin, notamment, d’anticiper les questions de réglementation et de protection des consommateurs. L’AMF s’intéresse de près à l’utilisation responsable de l’intelligence artificielle (IA) et mène une réflexion active sur ce sujet. L’AMF a récemment publié un document de réflexion et de discussion sur les meilleures pratiques pour l’utilisation responsable de l’IA dans le secteur financier. Un aperçu de ces bonnes pratiques sera présenté.
Comité scientifique | Scientific Comittee
- Marie-Pier Côté (Université Laval)
- Arthur Charpentier (UQÀM)
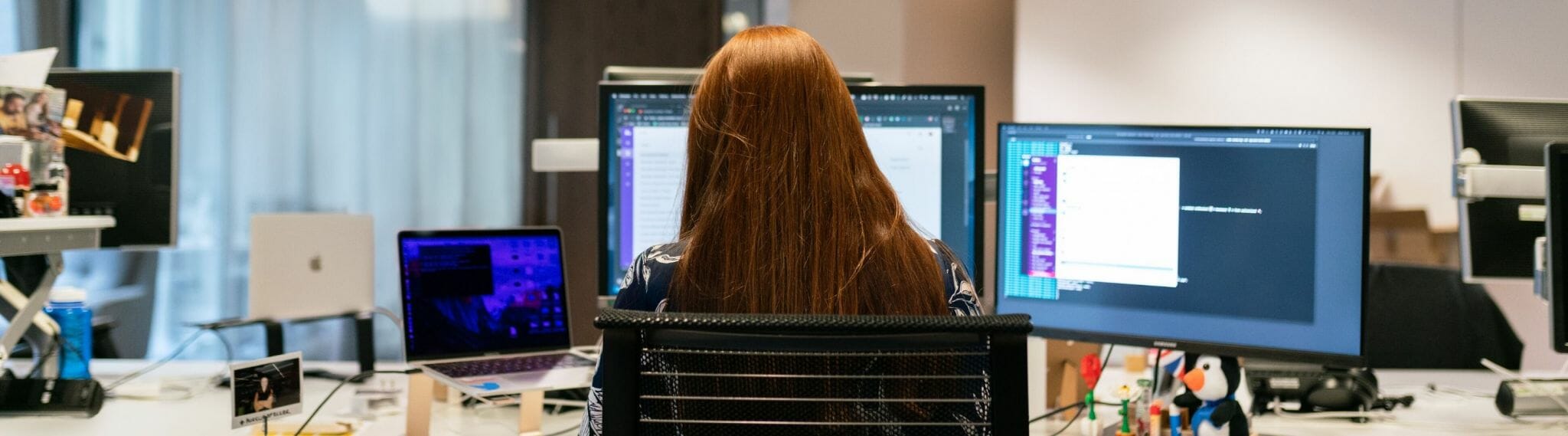
À propos de la Chaire de Leadership en enseignement en analyse de données massives pour l’actuariat – Intact de l’Université Laval
Les entreprises cherchent sans cesse de nouvelles façons de tirer parti des données afin de s’adapter au marché en évolution. Il est maintenant possible de collecter et d’analyser automatiquement des données de nombreuses sources: conversations avec les clients, clics dans les sites transactionnels, applications pour téléphones intelligents, capteurs liés à l’Internet des objets, etc. L’information contenue dans ces données faiblement structurées peut être transformée en un savoir stratégique, ouvrant la voie à des approches proactives et personnalisées pour chaque client.
Le nouveau paradigme des données massives crée des possibilités sans précédent pour les sociétés d’assurance: une meilleure segmentation des profils clients, une estimation plus précise du coût réel des produits pour une tarification améliorée, un suivi étroit de la satisfaction des clients, le développement de modèles dynamiques pour l’évaluation du passif des sinistres, etc. Cette chaire de leadership en enseignement (CLE) aide à améliorer la capacité d’analyse des données massives des actuaires et des sociétés d’assurance.
About the Chair in Eductional Leadership on big data analytics for actuarial sciences – Intact
Companies are constantly looking for new ways to leverage data to adapt to the changing marketplace. It is now possible to automatically collect and analyze data from many sources: conversations with customers, clicks on transactional sites, smartphone applications, sensors linked to the Internet of Things, and so on. The information contained in this loosely structured data can be transformed into strategic knowledge, paving the way for proactive, personalized approaches for each customer.
The new paradigm of massive data creates unprecedented opportunities for insurance companies: better segmentation of customer profiles, more accurate estimation of the real cost of products for improved pricing, close monitoring of customer satisfaction, development of dynamic models for assessing claims liabilities, etc. This Educational Leadership Chair help improve the capacity of actuaries and insurance companies to analyze massive data.
Restons en contact!
Vous souhaitez être informé des nouvelles et activités de l'IID? Abonnez-vous dès maintenant à notre infolettre mensuelle.