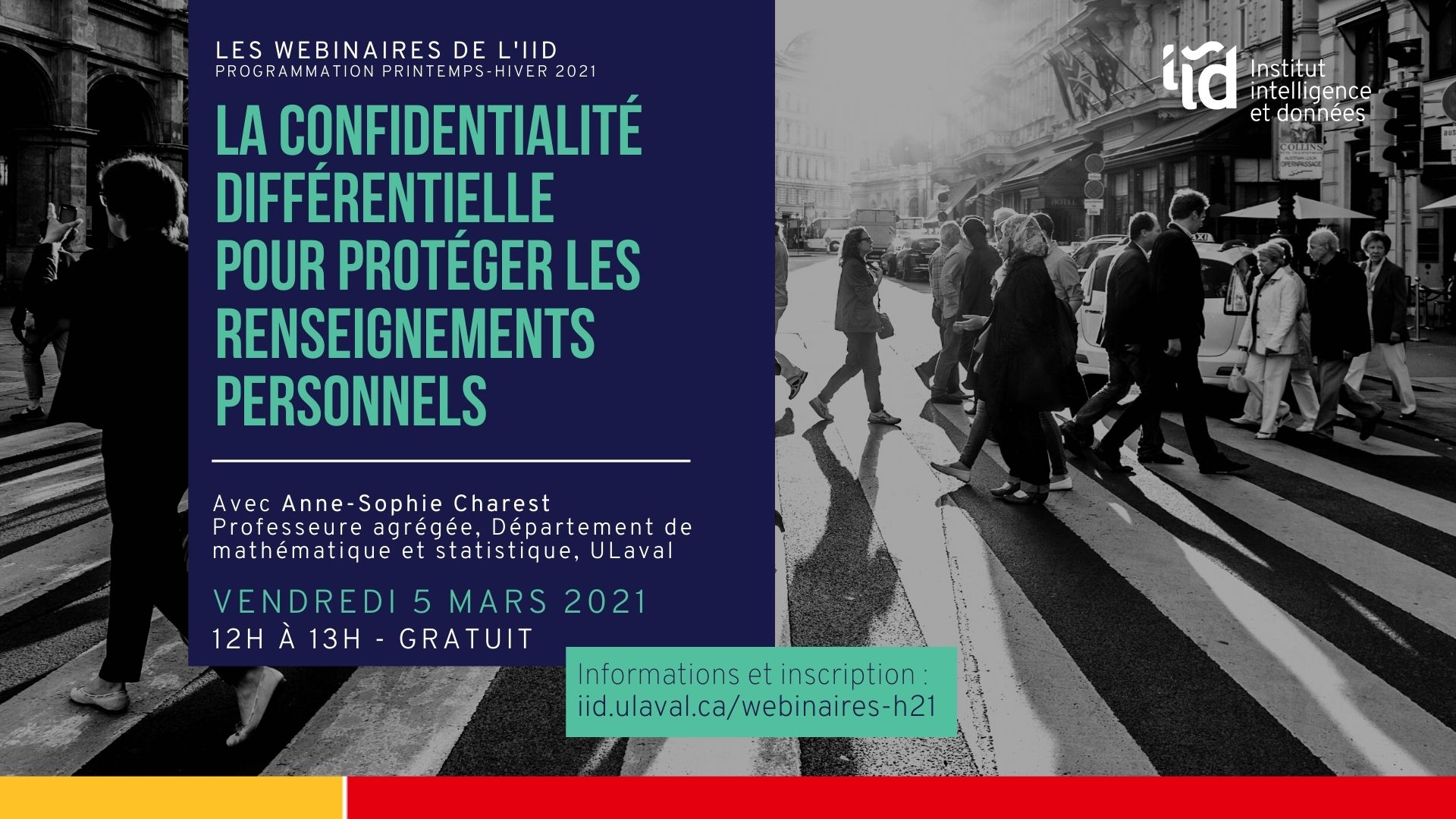
Differential confidentiality to protect personal information
Professor Anne-Sophie Charest offers a basic introduction to differential confidentiality, emphasizing the interpretation of measurement and the tools necessary for its implementation in different contexts.
Presentation Abstract
The purpose of differential confidentiality is to allow statistical analysis of a dataset without revealing the personal information of participants. To do this, it formally measures the impact on an individual who agrees to be part of a dataset from which certain statistics will be published. This is an approach that is gaining popularity both among researchers and in practice, and will be used by the Census Bureau for the release of data from the 2020 US Census.
Professor Anne-Sophie Charest offers a basic introduction to differential confidentiality, emphasizing the interpretation of measurement and the tools necessary for its implementation in different contexts.
Speaker Bio
Associate Professor, Faculty of Science and Engineering, Université Laval
Anne-Sophie Charest is an Associate Professor of Statistics in the Department of Mathematics and Statistics at Université Laval. She completed a BA Honors in Probability and Statistics at McGill University, Montreal, and received a Master’s and Ph.D. in Statistics from Carnegie Mellon University, Pittsburgh.
Her research focuses on protecting the confidentiality of statistical data, in particular in the context of a population survey or census. She is particularly interested in the generation and analysis of synthetic datasets as well as the measurement of disclosure risk, especially through differential confidentiality. She is a member of the Big Data Research Center at Université Laval.
Let’s keep in touch!
Would you like to be informed about IID news and activities? Subscribe now to our monthly newsletter.