Machine learning for the insurance industry
Using corporate data from SSQ, this project will lead to the creation of the most accurate, fair and equitable predictive models for clients’ needs in terms of insurance products and for certain aspects of their behaviour, such as the likelihood that a customer will not renew a particular insurance policy.
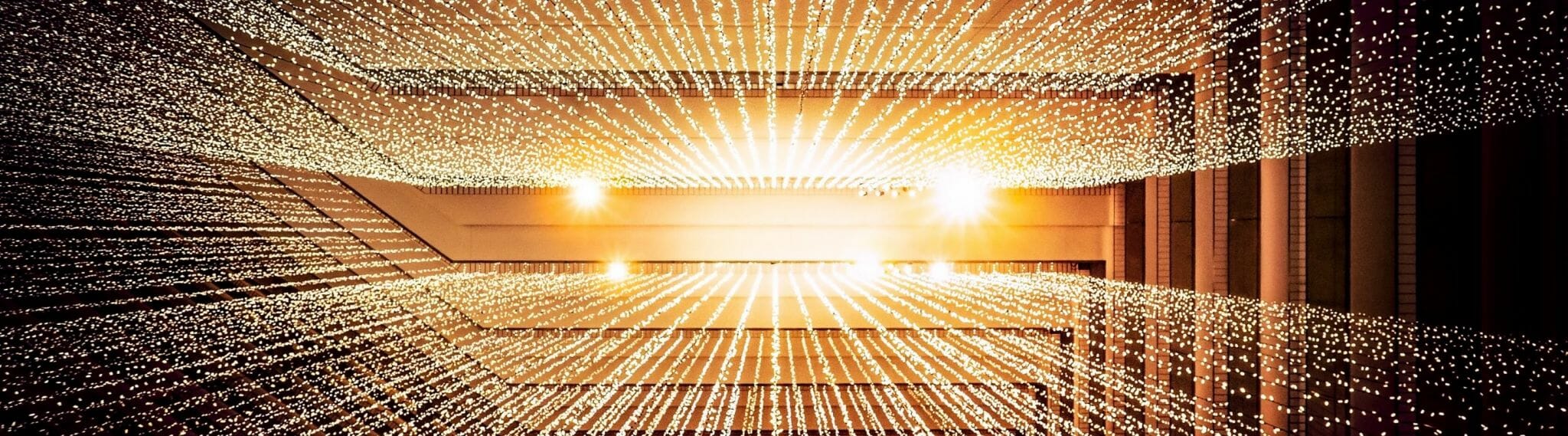
Predictive models, fraud detection and fairness: How can machine learning work for the insurance industry?
At the heart of their mission, the insurance industry strives to satisfy its customers and offer them the insurance products best suited to their needs. With the wealth of corporate data accumulated over the years, the availability of impressive computational resources, and the current state of knowledge in machine learning research, insurance companies can now strive to create effective predictive models for certain aspects of customer behaviour and their needs.
However, insurance companies are also accountable to our society and, in particular, this implies that they should not offer any service or coverage that is discriminatory in any way, in terms of race, skin colour, ethnicity or other irrelevant characteristics which are immoral to use. In this sense, the insurance industry should also be fair in the service it provides.
This research proposal aims to advance the current state of knowledge in areas of machine learning research of primary interest to the insurance industry.
More specifically, from SSQ’s corporate data, the research team aims to create the most accurate, fair and equitable predictive models for customer needs in terms of insurance products and for certain aspects of their behaviour, such as the likelihood that a customer will not renew a particular insurance policy.
The team also aims to set up accurate and fair fraud detectors capable of detecting fraud at an early stage and detecting new types of fraud.
To achieve these goals, the research team will need to adapt existing machine learning algorithms in innovative ways and design new ones, such that they can use and combine different data sources during learning, some of which are sequential in nature. Additionally, we will need to find ways to enforce fairness in machine learning algorithms, so that the predictors generated by these algorithms will not indirectly use sensitive attributes (such as race, ethnicity, religion, etc.) so as to make the algorithms perform unevenly among different groups of individuals.
Partner Organizations
Research Team
IID Principal Investigator of this project
IID Co-Investigators participating in the project
Project Team
Principal Investigator: Mario Marchand (Université Laval)
Co-Investigators: Thierry Duchesne (Université Laval) and Christian Gagné (Université Laval)
Project Funding: 2019-2023
Let’s keep in touch!
Would you like to be informed about IID news and activities? Subscribe now to our monthly newsletter.