Health+AI - techniques, applications, ethical and legal frameworks
Designed by specialists for non-specialists, this training course aims to equip users to assess the potential, challenges and limits of AI in healthcare, and to better evaluate or support its integration into current practices.
To support the development of knowledge about artificial intelligence (AI) and the use of data in healthcare, Université Laval’s Institut intelligence et données (IID) and its partners are offering this online training course.
Designed for non-specialists, this course explores AI tools and techniques, data management and stewardship, and the ethical and legal frameworks that support them.
The aim of this online training course is to equip users to assess the potential, challenges and limits of artificial intelligence in healthcare, and thus facilitate and support its integration into current practices.
With this online training course…
- You will understand the basic principles of artificial intelligence and data analysis;
- You will grasp the issues and best practices in the management and use of AI and data in healthcare;
- You will grasp the technical concepts and mechanisms of AI;
- You will understand the ethical, regulatory and normative frameworks governing the use of healthcare data;
- You will discover examples of digital innovation in healthcare.
Training offered online. Duration: 10h
Who should attend?
Developed by experts, this course is aimed at anyone interested in and concerned by the use of data and AI systems in healthcare and related fields, whether in research (students, researchers, professionals), practice (professionals, practitioners) or administration (managers).
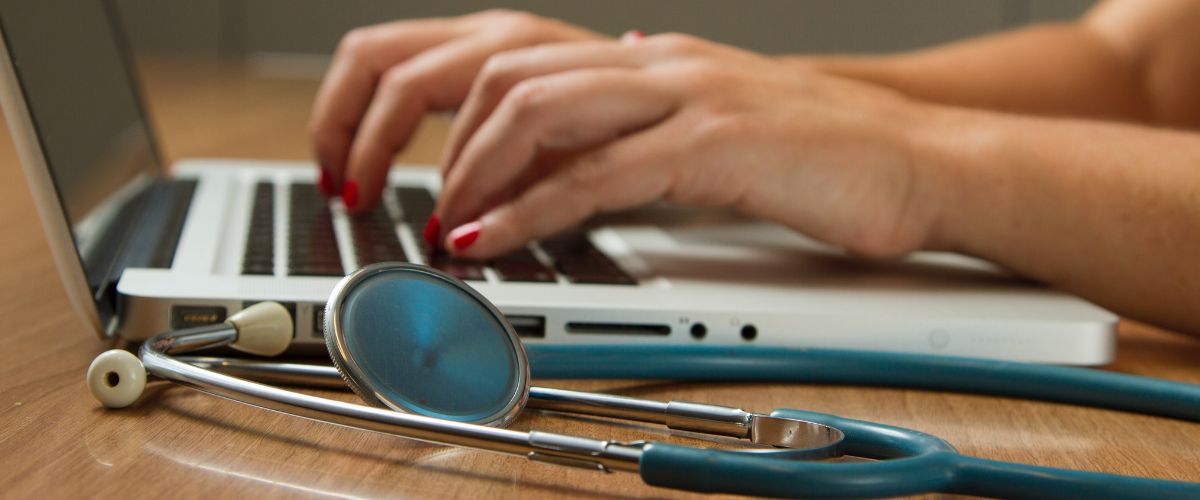
The training at glance
Module 1 / The ABC of AI
This first introductory module briefly presents the concepts of artificial intelligence and digital intelligence: what it is in concrete terms, what it can do, why and how to use it. It provides a definition of AI in healthcare, and what an AI system for healthcare is.
Module 2 / Data in healthcare
This second module provides an overview of the issues and realities associated with the use of data, both in research and in practice, in health-related fields. It offers a general understanding of the critical importance of data and its use in Quebec’s healthcare sector.
After an initial dive into the world of healthcare innovation and the key issues surrounding the use of data, the focus shifts to understanding the data generated by learning healthcare systems. We discover the life cycle of data and its essential role in achieving the objectives of a learning healthcare system.
The importance of good practice in the use of data in the context of digital health and artificial intelligence is then presented. The adoption of FAIR principles and data curation are discussed, before focusing on data interoperability, data representation standards and the improvements needed to facilitate their use.
Finally, the module concludes with an exploration of the current limits and prospects of artificial intelligence in Quebec healthcare. We discover the challenges that need to be met to fully exploit the potential of AI in the healthcare system.
Module 3 / Technical concepts
The third module aims to provide non-experts with a basic knowledge of the technical concepts of machine learning, deep learning and their applications, while highlighting the specific challenges and strategies of AI in healthcare.
After an initial overview of AI and its evolution, the basic notions of machine learning and the vocabulary essential for understanding more advanced concepts are presented. Some examples of supervised and unsupervised learning algorithms are explored. It then focuses on deep learning, its neural network structure and the model training process.
Various applications of deep learning are then outlined, particularly in the fields of computer vision and sequential data processing, with a discussion of how they work, their architectures and concrete examples. The importance of model evaluation and metrics such as accuracy, precision and specificity is then examined, before turning to the concepts of underlearning and overlearning.
Finally, the module concludes with a discussion of the challenges, strategies and limitations of AI for healthcare, around concepts such as the distinction between causation and correlation, the interpretability and explicability of models, the generalizability and robustness of healthcare data, as well as its sensitivity and confidentiality.
Module 4 / Ethics and regulation
This fourth module aims to provide an in-depth understanding of the ethical, legal and regulatory issues surrounding the use of data and AI in healthcare, while highlighting the importance of responsible governance of healthcare data to ensure the protection of individuals.
We begin by exploring the fundamental concepts of ethics and the different modes of regulation, assessing their advantages and shortcomings. We then turn to the ethics of governance in the context of health and AI, highlighting the ethical and legal issues associated with the analysis and valorization of health data.
This is followed by an exploration of the ethical issues, frameworks and principles involved in the use of AI and data in healthcare. In particular, it examines how ethical charters can be used to formalize ethical principles. This is followed by an examination of the legal frameworks governing the use of health data in Quebec, including access and secondary use of data, as well as the impacts of Bill 25 on research.
Finally, the module addresses the regulation of software as medical devices, particularly those using machine learning algorithms. It examines the risks, regulatory implications and best practices for the development of machine learning algorithms in this context.
Module 5 / Digital innovation in healthcare
This final module explores some examples and opportunities for innovation linked to the use of health data and AI in the medical field.
First, the importance of involving patients and citizens in projects using their health data, particularly those involving AI, is discussed. It presents various methods, best practices and concrete examples illustrating how this engagement can be implemented. It then explores the Learning Health Systems (LHS) approach, and the iterative learning cycle required to operationalize it. Finally, the module looks at collaborative writing applications in the context of learning healthcare systems.
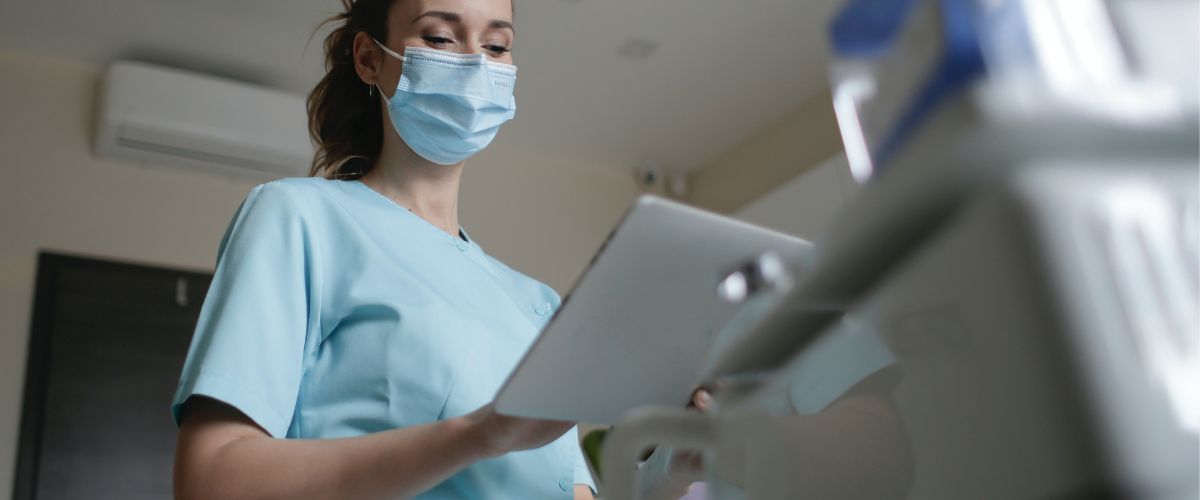
List of trainers (in alphabetical order)
- Lyna Abrougui, MsC candidate in computer science, Université Laval
- Sylvain Auclair, PhD candidate in philosophy, Université Laval
- Patrick Archambault, researcher-clinician, Centre de recherche de l’Hôtel-Dieu de Lévis
- Frédéric Beaupré, PhD student in biophotonics, Université Laval
- Tess Berthier, coordinator, Plateforme IA-Santé PREDIS
- Camille Besse, data scientist, Institut intelligence et données (IID)
- Anthony Bilodeau, PhD student in biophotonics, Université Laval
- Catherine Bouchard, PhD student in electrical engineering, Université Laval
- Antoine Boudreau-Leblanc, PhD candidate in bioethics, Université de Montréal
- Audrey Durand, assistant professor, Faculté des sciences et de génie de l’Université Laval
- Simon Duchesne, professor, Faculté de médecine de l’Université Laval
- Christian Gagné, director, Institut intelligence et données (IID)
- Jean-Baptiste Gartner, candidate for PhD in management, Université Laval
- Raphaëlle Giguère, candidate for MsC in computer science, Université Laval
- Géraldine Layani, clinical assistant professor, Département de médecine de famille et médecine d’urgence de l’Université de Montréal
- Jean-Noël Nikiema, assistant professor, École de santé publique de l’Université de Montréal
- Félix-Arnaud Morin-Bertrand, research professional, Faculté des sciences sociales de l’Université Laval
- Cécile Petitgand, data management consultant, Centre de recherche du CHUM
- Louise Ringuette, PhD candidate in bioethics, Université de Montréal
- Bryn Williams-Jones, professor, École de santé publique de l’Université de Montréal
This training course is offered on the BRIO platform by Université Laval’s Institut intelligence et données (IID), thanks to the financial support of the Ministère de l’Économie, de l’Innovation et de l’Énergie through its NovaSciences program.
Let’s keep in touch!
Would you like to be informed about IID news and activities? Subscribe now to our monthly newsletter.